Information Box Group
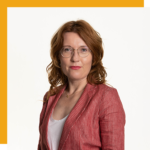
Dr. Ivona Kučerová Learn More
Director, ARiEAL
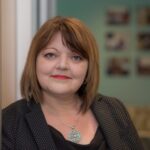
Dr. Anna Moro Learn More
Associate Director, ARiEAL
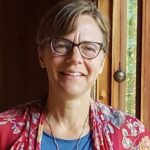
Dr. Sue Becker Learn More
Member of ARiEAL
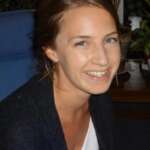
Dr. Alison Biggs Learn More
Member of ARiEAL
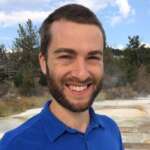
Dr. Christian Brodbeck Learn More
Member of ARiEAL
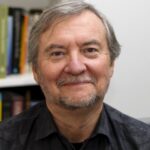
Dr. John Connolly Learn More
Member of ARiEAL
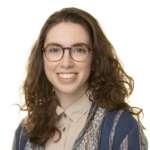
Dr. Lauren Fink Learn More
Member of ARiEAL
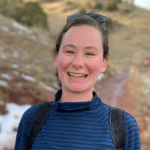
Dr. Phoebe Gaston Learn More
Member of ARiEAL
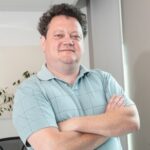
Dr. Victor Kuperman Learn More
Member of ARiEAL
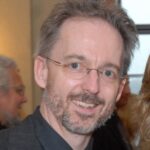
Dr. Michael Noseworthy Learn More
Member of ARiEAL
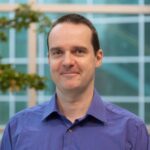
Dr. Daniel Pape Learn More
Member of ARiEAL
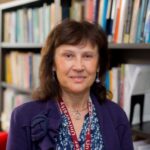
Dr. Elisabet Service Learn More
Member of ARiEAL
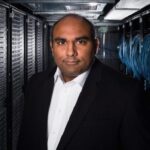
Dr. Ranil Sonnadara Learn More
Member of ARiEAL
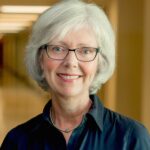
Dr. Lyn Turkstra Learn More
Member of ARiEAL
Past Members
Dr. Catherine Anderson is an associate professor at the Department of Linguistics and Languages at McMaster University and was a member of ARiEAL until 2022. Dr. Anderson directed the Teaching and Learning Lab. The lab’s research, all of which is conducted in collaboration with undergraduate student partners, focused on students’ experiences in a variety of undergraduate learning contexts.
Dr. Lisa Archibald was a member of ARiEAL until 2022 as the director of the The Language and Working Memory Lab, which is part of the School of Communication Sciences and Disorders at The University of Western Ontario. The Language and Working Memory Lab focuses on investigating how language and memory processes interact in both children learning at a typical rate and those with learning disabilities.
Professor Carol DeMatteo was a member of ARiEAL until 2022. As a clinician scientist, Professor DeMatteo specializes in childhood neurotrauma, specifically acquired brain injury including concussion. Professor DeMatteo officially retired in 2021 and has since assumed the rank of Professor Emeritus at McMaster University. Yet, she continues with her concussion research at CanChild Centre for Childhood Disability Research.
Dr. Marc Joanisse was a member of ARiEAL until 2022. He directs the Language, Reading and Cognitive Neuroscience Lab which is housed in The University of Western Ontario’s Brain and Mind Institute. Dr. Joanisse’s research focuses on the cognitive and neural foundations of language and reading across the lifespan. This includes studying reading and language disorders in children, as well as language learning and processing in adults.
Professor Reilly is an internationally renowned researcher in the field of signal processing. He has over 35 years of experience in applying signal processing methods to difficult problems in industry and medicine. Altogether he has 8 patents for which he is the principal inventor.
Recently, Professor Reilly’s major research focus has been on the application of machine learning principles to the analysis of various forms of brain imaging modalities, primarily the EEG. His team was the first to apply rigorous machine learning analysis of EEG signals to predict the response of an individual patient to various forms of treatment for major depression. Also with Professor John Connolly and Dr. Narges Armanfard, his team developed an EEG-based automated machine learning approach for determining the prognosis for recovery of coma patients.
Email: reillyj@mcmaster.ca
Representative Publications:
Pedro L. Ballester, Jee Su Suh, James P. Reilly, Benicio N. Frey, (2023). “Gray matter volume and ventricle size drive the brain age gap in schizophrenia: A SHAP study”, Schizophr, 9(3)
Devon Watts, Diego Librenza-Garcia, Pedro Ballester, Ives Passos, Felix Kessler, Luiza Gea, James Reilly, Flavio Kapczinski, Gary Chaimowitz, (2022) “Predicting criminal and violent outcomes in psychiatry: a meta-analysis of diagnostic accuracy“, Translational Psychiatry 12, 470
Watts, D., Pulice, R.F., Reilly, J. et al. (2022) Predicting treatment response using EEG in major depressive disorder: A machine-learning meta-analysis. Transl Psychiatry 12, 332
David Prete, David Heikoop, Josh McGillivray, James Reilly and Laurel J.Trainor, (2022) “The sound of silence: Predictive error responses to unexpected sound omission in adults”, European Journal of Neuroscience 55(8), pp. 1972 – 1985
Anastasiya Slyepchenko, Luciano Minuzzi, James P. Reilly, Benicio N. Frey, (2022) “Longitudinal Changes in Sleep, Biological Rhythms and Light Exposure from Pregnancy to Postpartum and Their Impact on Perinatal Mood and Anxiety”, The Journal of Clinical Psychiatry 83 (2), p39211.
Colic S, He JC, Richardson JD, Cyr KS, Reilly JP, Hasey GM. (2022) “A machine learning approach to identification of self-harm and suicidal ideation among military and police Veterans”. Journal of Military, Veteran and Family Health, 8 (1), pp 56 – 67.
Masychev, K., Ciprian, C., Ravan, M., Reilly, J. P., & MacCrimmon, D. (accepted). A Machine Learning Approach Using Effective Connectivity to Predict Response to Clozapine Treatment. IEEE Transactions on Neural Systems and Rehabilitation Engineering.
Masychev, K., Ciprian, C., Ravan, M., Reilly, J. P., & MacCrimmon, D. (2021). Advanced Signal Processing Methods for Characterization of Schizophrenia. IEEE Transactions on Biomedical Engineering, 68(4), 1123-1130.
Boshra, R., Ruiter, K. I., DeMatteo, C., Reilly, J. P., & Connolly, J. F. (2019). Neurophysiological correlates of concussion: Deep Learning for clinical Assessment. Scientific reports, 9(1), 1-10.
Shaw, S., Dhindsa, K., Reilly, J., Becker, S. (2019). Capturing the forest but missing the trees: Microstates inadequate for characterizing shorter-scale EEG dynamics, Neural Computation, 31(11), 2177-2211.
Chrapka, P., de Bruin, H., & Reilly, J. (2019). Estimating Neural Sources Using a Worst-Case Robust Adaptive Beamforming Approach. Biomedical Signal Processing and Control, 52, 330-340.
Chrapka, P., de Bruin, H, Hasey, G. & Reilly, J. (2019). Wavelet-based Muscle Artifact Noise Reduction for Short Latency rTMS Evoked Potentials. IEEE Transactions on Neural Systems and Rehabilitation Engineering, 27(7), 1449-1457.
Connolly, J. F., Reilly, J. P., Fox-Robichaud, A., Britz, P., Blain-Moraes, S., Sonnadara, R., Hamielec, C., Herrera-Diaz, A., & Boshra, R. (2019). Development of a point of care system for automated coma prognosis: a prospective cohort study protocol. BMJ Open, 9, e029621.
Boshra, R., Dhindsa, K., Boursalie, O., Ruiter, K. I., Sonnadara, R., Samavi, R., Doyle, T. E., Reilly, J. P., & Connolly, J. F. (2019). From Group-Level Statistics to Single-Subject Prediction: Machine Learning Detection of Concussion in Retired Athletes. IEEE Transactions on Neural Systems and Rehabilitation Engineering, 27(7), 1492–1501.
Armanfard, N., Komeili, M., Reilly, J. P., & Connoly, J. (2019). A Machine Learning Framework for Automatic and Continuous MMN Detection with Preliminary Results for Coma Outcome Prediction. IEEE Journal of Biomedical and Health Informatics, 23(4), 1794-1804.
Armanfard, N., Reilly, J. P., & Komeili, M. (2017). Logistic Localized Modeling of the Sample Space for Feature Selection and Classification. IEEE Transactions on Neural Networks and Learning Systems, 1–18.
Armanfard, N., Reilly, J. P., & Komeili, M. (2016). Local Feature Selection for Data Classification. IEEE Transactions on Pattern Analysis and Machine Intelligence, 38(6), 1217–1227.
Armanfard, N., Komeili, M., Reilly, J. P., Mah, R., & Connolly, J. F. (2016). Automatic and Continuous Assessment of ERPs for Mismatch Negativity Detection. 2016 38th Annual International Conference of the IEEE Engineering in Medicine and Biology Society (EMBC) Proceedings, 969–972.
Ravan, M., Hasey, G., Reilly, J. P., MacCrimmon, D., & Khodayari-Rostamabad, A. (2015). A machine learning approach using auditory odd-ball responses to investigate the effect of Clozapine therapy. Clinical Neurophysiology, 126(4), 721–730.
Harrison, A. H., Noseworthy, M. D., Reilly, J. P., & Connolly, J. F. (2014). Ballistocardiogram correction in simultaneous EEG/ fMRI recordings: a comparison of average artifact subtraction and optimal basis set methods using two popular software tools. Critical Reviews in Biomedical Engineering, 42(2), 95–107. Retrieved from
Ravan, M., Reilly, J. P., & Hasey, G. (2014). Minimum variance brain source localization for short data sequences. IEEE Transactions on Biomedical Engineering, 61(2), 535–546.
Hasey, G., Khodayari-Rostamabad, A., MacCrimmon, D., De Bruin, H., & Reilly, J. P. (2014). Expert System For Determining Patient Treatment Response. US Patent 8655817.
Khodayari-Rostamabad, A., Reilly, J. P., Hasey, G. M., de Bruin, H., & MacCrimmon, D. J. (2013). A machine learning approach using EEG data to predict response to SSRI treatment for major depressive disorder. Clinical Neurophysiology, 124(10), 1975–1985.
Ravan, M., Reilly, J. P., Trainor, L. J., & Khodayari-Rostamabad, A. (2011). A machine learning approach for distinguishing age of infants using auditory evoked potentials. Clinical Neurophysiology, 122(11), 2139–2150.
Khodayari-Rostamabad, A., Hasey, G. M., MacCrimmon, D. J., Reilly, J. P., & Bruin, H. De. (2010). A pilot study to determine whether machine learning methodologies using pre-treatment electroencephalography can predict the symptomatic response to clozapine therapy. Clinical Neurophysiology, 121(12), 1998–2006.
Rahbar, K., & Reilly, J. P. (2005). A frequency domain method for blind source separation of convolutive audio mixtures. IEEE Transactions on Speech and Audio Processing, 13(5), 832–844.